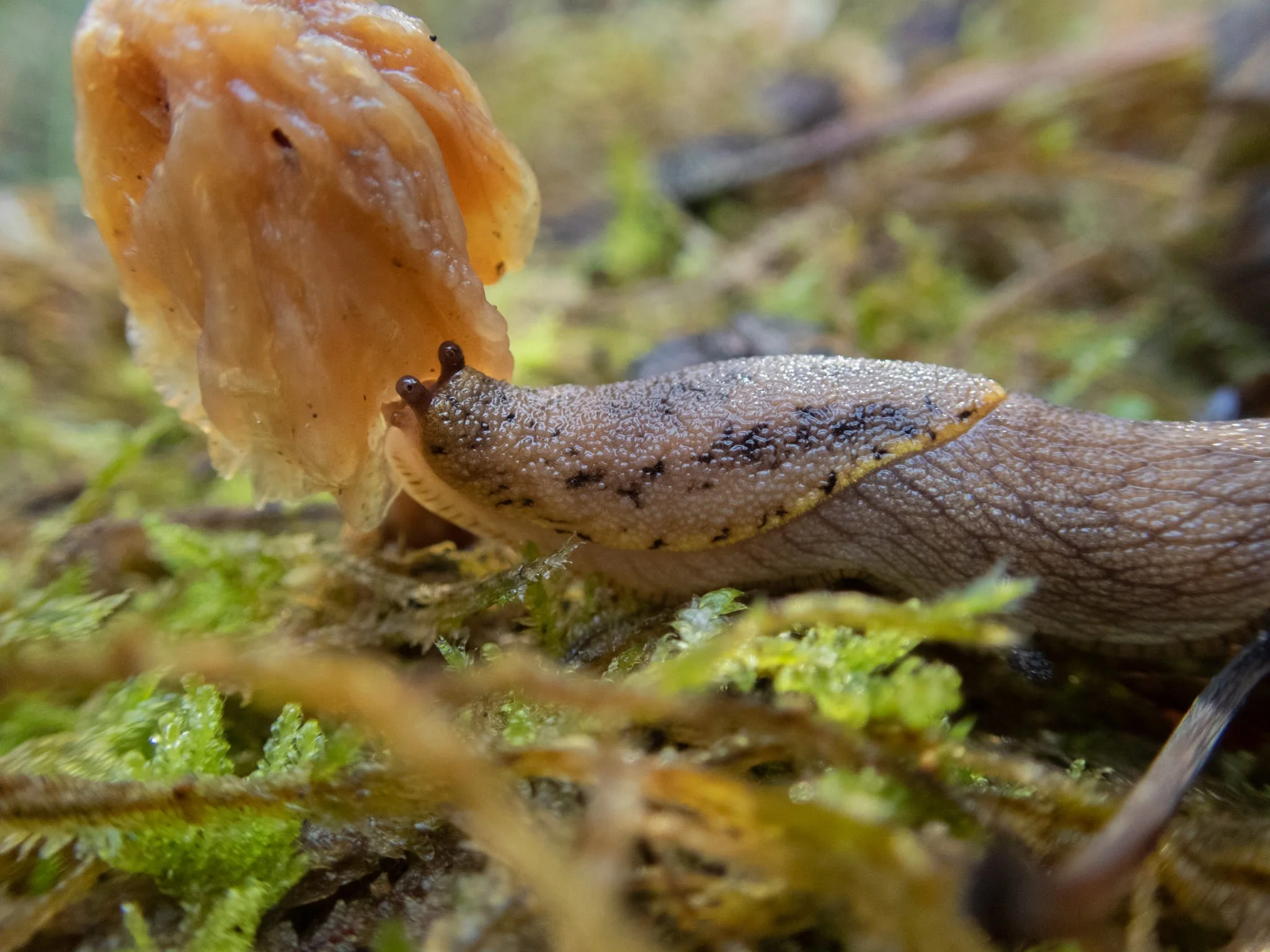
Research
Speciation in tail-dropper slugs
Figure 1: Sister species P. andersoni and P. foliolatum have partially overlapping ranges and differ in ecologically important traits, like microhabitat, foot size, and dentition. A) Map of the Pacific Northwest showing glacial extent during the Last Glacial Maximum (blue), the range of P. andersoni (orange), and the range of P. foliolatum (purple). B) P. andersoni and C) P. foliolatum feeding on mushrooms. D) Comparisons of dentition between P. andersoni and P. foliolatum (Pilsbry & Vanatta 1898).
Taildropper slugs (genus Prophysaon) are endemic to the temperate rainforests of the Pacific Northwest (Figure 1A). There are nine described species, and the group appears to have a complex history with the potential for geology, climate, and ecology to have driven diversification. During my dissertation, I developed a novel approach to delimit species while considering population-level processes (delimitR) and inferred a likely history of divergence in isolated refugia during glaciation, followed by expansion and gene flow between lineages upon secondary contact in several species (Smith & Carstens 2020, Smith et al. in review). We are currently collecting genomic and transcriptomic to learn not only whether and when gene flow has occurred between species, but also which regions of the genome have introgressed across species and population boundaries. We’re also using Scanning Electron Microscopy (SEM) to image the radula (i.e. dentition) of these slugs in an attempt to quantify phenotypic variation in an ecologically relevant trait (Figure 2).
Figure 2: The radula of a specimen of Prophysaon foliolatum. The radula was dissected out and then imaged on a Scanning Electron Microscope at Indiana University.
Machine learning in population genetics and phylogenetics
Machine learning approaches are increasingly being applied to answer interesting questions in population genetics and phylogenetics. During my dissertation, I developed delimitR, an approach to delimit species in the presence of population-level processes using machine learning and genomic data. In ongoing work, we are evaluating the power of machine learning approaches to incorporate complex processes, like background selection, that may mislead traditional population genetics approaches. We are also interested in applying machine learning to questions in phylogenetics.
Accounting for heterogeneity in phylogenomics
Figure 3: Gene duplication and loss can lead to gene tree heterogeneity. After duplication, two copies evolve (green and pink). Gene copies can be orthologous (i.e. share a common ancestor due to speciation), or paralogous (i.e. share a common ancestor due to duplication).
Increasing evidence points to rampant heterogeneity across the genome. In other words, gene histories often disagree with each other and with the species history. Processes generating this heterogeneity include gene duplication and loss (Figure 3), incomplete linage sorting, and introgression. In previous research, I have investigated the potential benefits and risks of using paralogs (genes related through duplication events) for phylogenetic inference (Yan et al. 2021; Smith and Hahn 2021; Smith and Hahn 2022; Smith et al. 2022). This work highlighted the robustness of phylogenetic inference to the heterogeneity introduced by the inclusion of paralogs and suggests steps towards including more data in phylogenetic analyses. Our ongoing research aims to integrate diverse datatypes and processes into frameworks for phylogenetic inference.
The field of comparative phylogeography aims to understand how communities of different species respond to geologic and climatic events. While inferring whether individual species have responded similarly or idiosyncratically is of interest, the ultimate goal is to better understand the factors that drive species responses to environmental change, and this requires an integration across genetic, ecological, and phenotypic data. Predictive phylogeography leverages data collected across taxa to make predictions about unstudied taxa and to identify factors predictive of species’ responses. As with any predictive model, our predictions are only as good as the data used to build the model. Our research aims to increase taxon sampling for predictive phylogeography using traditional (Figure 4) and novel (e.g., environmental DNA) approaches.
Using predictive phylogeography to study community responses to environmental change
Figure 4: Sampling of leaf litter and micro-invertebrates from the Pacific Northwest of North America in 2017. A) Map of sampling localities across the Pacific Northwest. NC: North Cascades, BW: Blue and Wallowa Mountains, SC: South Cascades, NRM: Northern Rocky Mountains, VI: Vancouver Island. B) Pie chart showing the classes of the sequenced invertebrates, based on BLASTn results. C) Photograph of a Punctum randolphii sample. D) Photograph of Columella edentula sample.